WS 06: ISDAEB – Information System Design, Analytics & Economic Behavior
Workshop Beschreibung
Information systems (IS) have evolved to business enablers that fundamentally change the (economic) behavior of market participants. “Enabling IS” mediate interactions among individuals and organizations by analyzing (customer) data, aggregating data and preferences, and coordinating transactions and resource allocation. Examples include electronic auction markets, crowd sourcing platforms, online labor markets, reputation mechanisms, e-voting systems, or recommender systems.
Designing “enabling IS” requires an in-depth understanding of how humans and organizations interact with each other, and how the parameters of a decision situation shape its outcome. Game theory, experimental and behavioral economics provide this understanding in the form of fundamental models about the equilibrium behavior of players in complex strategic environments. They offer valuable guidelines for modelling the behavioral patterns of humans, organizations and society and for IS design. For example, game theory and experimental economics are central to the design of ad auctions, sharing platforms, and reputation mechanisms. Economic theory provides the basis for the design of policy measures to mitigate negative externalities created through “enabling IS” such as sharing platforms.
Beyond implementing economic models, designing “enabling IS” poses new challenges and creates new insights that feed back into theory: IS designers often face utility functions and design desiderata that are quite different from the ones that are described in economic textbooks. “Enabling IS” allow for the implementation and the evaluation of completely new designs that have not yet been described in the theoretical literature. Data generated by “enabling IS” in combination with advanced analytics methods and innovative algorithms promise to deliver unexpected insights and detect unknown behavioral patterns, which in turn enrich economic theory and inform the design of “enabling IS”.
This workshop series focuses on research at the various interfaces between IS Design, Analytics & Economic Behavior. The workshop welcomes all researchers who typically attend conferences such as WISE, CIST, TEIS, and the Economics of IS tracks at ICIS, ECIS etc. The workshop will feature up to four invited presentations in a single track. Each presentation includes a discussant and an intense plenary session. Generously planned time slots give room for in-depth, constructive academic debate.
Vorgesehene Zielgruppe: Wissenschaft
Datum des Workshops
Donnerstag 21.09.2023, ab 10:30 bis open end
Ablauf
10:30 – Ram Gopal, University of Warwick: Enhancing the Attractiveness of Online Lending for Lenders, Borrowers, and the Platform
Although online lending enjoyed explosive growth in the past decade, its market size remains small compared to other financial assets. The risk of losing money, stringent government regulations, and low awareness of the benefits have hampered the realization of the full potential of the online lending market. Because online loans are an emerging asset class, investors may not be aware of the investment performance of online loans compared to other assets, and it remains an open question whether online loans offer sufficiently attractive returns to warrant inclusion in an asset allocation decision. To attract lenders, platforms must provide an appealing investment opportunity which entails construction of portfolios of loans that investors find attractive. We propose general characteristics-based portfolio policies (GCPP), a novel framework to overcome the difficulties associated with portfolio construction of loans. GCPP directly models the portfolio weight of a loan as a flexible function of its characteristics and does not require direct estimation of the distributional properties of loans. Using an extensive dataset spanning over one million loans from 2013 to 2020 from LendingClub, we show that GCPP portfolios can achieve an average annualized internal rate of return (IRR) of 8.86% to 13.08%, significantly outperforming an equal-weight portfolio of loans. We then address the question of whether online loans can earn competitive rates of return compared to traditional investment vehicles through six market indices covering stocks, bonds, and real estate. The results demonstrate that a portfolio of online loans earns competitive or higher rates of return compared to traditional asset classes. Furthermore, the IRRs of the loan portfolios have small correlations with the benchmark index IRRs, pointing toward significant diversification benefits. Together, we demonstrate that GCPP is an approach that can help platforms better serve both borrowers and lenders en route to growing their business.
Our work naturally leads to the question of how interest rates of unsecured personal loans be set. Much of the literature advocates risk-based pricing: A loan with a high degree of risk should be assigned a high interest rate, and a loan with low risk should be assigned a low interest rate. Risk-based pricing has also been widely applied to the loan market in practice. However, the existing literature does not consider the economic rationale that interest rates should provide the same risk-return tradeoff, which results in the mispricing that risky borrowers pay a much larger premium compared to less risky borrowers. Employing GCPP, we find that the interest rates on the platform are not always set appropriately. Our results show that the price distortion leads to a significant gap in the probability of being funded between subprime (660<FICO<700) and prime (FICO>700) borrowers. We also provide evidence consistent with a bias against African Americans in setting interest rates.
We provide an alternative set of interest rates aimed at alleviating mismatches in risk and return. These interest rates lead to greater credit allocation to underserved subprime borrowers, narrowing the gap between subprime and prime borrowers. The new interest rates assigned by our method also reduce biases against minority groups, such that two borrowers with similar characteristics but different races are assigned similar interest rates. Finally, when interest rates are appropriately set, the platform benefits. LendingClub derives its revenue from the number of users. Maximizing revenue is equivalent to maximizing the volume of loans. Under the new interest rates, the loan volume is expected to increase by 15.5% compared to the volume under the original interest rates. Hence, online lending platforms can do well by doing good – maximizing revenue while providing more attractive loan pricing for both borrowers and lenders.
12:00-13:00 – Mittagspause
13:00 – Oliver Müller, University of Paderborn: Diagnosing and Mitigating Shortcut Learning in ML-Based IS Research
Machine learning (ML) methods have become widespread in information systems (IS) research. However, several methodological pitfalls in ML-based research have become known recently. An issue that warrants our special attention is the phenomenon of shortcut learning. Shortcuts are decision rules that perform well on standard benchmark datasets but fail to generalize to more challenging situations. Consequently, ML models that rely heavily on shortcuts fail when making inferences using data only slightly different from their original training data. Shortcut learning represents a severe threat to the reproducibility of ML-based studies and the generalizability of research results to real-world contexts. This article examines the danger of shortcut learning in ML-based IS research theoretically and empirically. Using simulated and real data, we demonstrate that shortcut learning can lead to dramatic overestimations of the generalization capabilities of ML models. Specifically, we found that the out-of-sample predictive performance of ML models suffering from shortcuts falls by up to 195 percent as testing conditions become more challenging and realistic. Besides proposing sampling strategies to identify shortcut learning, we present a set of guidelines for increasing the robustness of ML models and empirically demonstrate their effectiveness.
14:30-15:00 – Kaffeepause
15:00 – Sameer Mehta, Erasmus University Rotterdam: Pay with Your Data: Designing Optimal Data-Sharing Mechanisms for Artificial Intelligence Services
The rapid advances in the field of Machine Learning (ML) have led to the proliferation of Artificial Intelligence (AI) services offered by firms. To develop a valuable AI service, a firm must build an accurate ML model which, in turn, requires a large amount of training data. Present-day firms usually obtain this data by offering incentives to consumers to share their data during the initial development phase of the AI service, and then use that data to re-train the ML models to improve the quality of the AI service. Consumers, on the other hand, incur costs in sharing sensitive data. In this paper, we analyze two popular data-sharing mechanisms employed by firms: manual data-sharing and algorithmic data-sharing. In the former approach, consumers decide the amount of data to share with the firm, whereas in the latter, the firm develops a data-redaction algorithm – which identifies sensitive segments of data and redacts them – that decides the amount of data to collect from consumers. For both approaches, we obtain revenue-maximizing mechanisms for the firm and analyze the fundamental differences between the two approaches in terms of the revenue accrued by the firm, the consumer surplus, and the volume of data collected. We also analyze a hybrid mechanism where the firm offers both the data-sharing approaches and consumers self-select their choice. While the firm naturally obtains higher revenue under an optimal hybrid mechanism relative to the manual and algorithmic counterparts, we show that consumers may prefer the individual mechanisms. We examine the relative strengths of the mechanisms and identify real-world scenarios where the firm can deploy them.
Registration
ISDAEB 2023 is free of charge, but a registration is required – either here: https://soscisurvey.wu.ac.at/isdaeb2023/ or during the registration process for WI2023: https://wi2023.de/en/registration-conftool/
Organisatoren
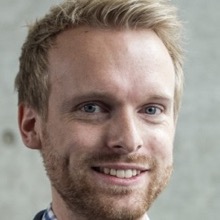
Alex Kupfer
Universität Innsbruck, Alexander.Kupfer(at)uibk.ac.at
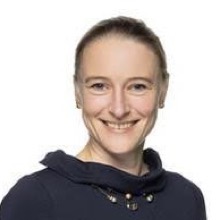
Verena Dorner
Wirtschaftsuniversität Wien, verena.dorner@wu.ac.at
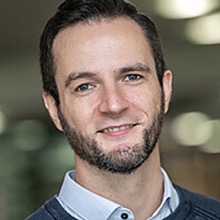
Dominik Gutt
Erasmus University Rotterdam, gutt@rsm.nl
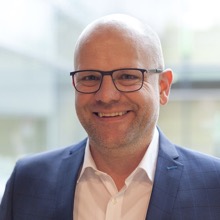
Steffen Zimmermann
Universität Ulm, steffen.zimmermann(at)uni-ulm.de